In today’s fast-paced digital world, safeguarding sensitive data has become a top priority, especially as data is often likened to ‘the new oil.’ With machine learning becoming a part of our daily lives, from suggesting music to diagnosing illnesses, there’s a growing need for preserving privacy. That’s where zero-knowledge machine learning (ZKML) comes into play. ZKML is a groundbreaking approach that ensures both the effective use of data and keeping it confidential. In this article, we’ll delve into the essence of Zero-knowledge machine learning (ZKML), explore how it operates, discuss its implications, and ponder the potential future developments in this field.
Zero-knowledge machine learning (ZKML) is all about striking a balance between data utility and maintaining confidentiality. As it becomes more deeply integrated into our lives, it’s crucial to understand how this technology functions and its far-reaching effects. We’ll unpack how ZKML works, its real-world implications, and consider the exciting possibilities it might offer in the future. So, let’s embark on this journey to demystify the world of Zero-knowledge machine learning (ZKML) and discover the role it plays in the ever-evolving landscape of data privacy and machine learning.
What is Zero-Knowledge Machine Learning (ZKML)?
Zero-Knowledge Machine Learning (ZKML) is a clever blend of two important fields: machine learning and zero-knowledge proofs. Essentially, it lets us use data to train machine learning models without ever giving away the actual data. This comes in handy, especially when we’re dealing with sensitive information, like personal medical records or financial data. Instead of sending or showing the raw data, Zero-knowledge machine learning (ZKML) allows us to prove that the data is valid and accurate without revealing the actual details.
In more straightforward terms, Zero-knowledge machine learning (ZKML) is like a secret handshake for data. It enables us to share information without exposing the nitty-gritty details. Think of it as a secure way to make sure your data is being put to good use without giving it all away. So, in a world where privacy and security are paramount, Zero-knowledge machine learning (ZKML) is an exciting development that keeps our sensitive information safe while still letting machines learn and make useful predictions.
The Mechanics Behind ZKML
Zero-Knowledge Machine Learning (ZKML) is an emerging concept that combines two important fields: zero-knowledge proofs and machine learning. Here’s some context on the mechanics behind ZKML:
Zero-Knowledge Proofs
Zero-knowledge proofs are cryptographic protocols that allow one party (the prover) to prove to another party (the verifier) that they possess certain information without revealing the information itself. In essence, it allows one party to prove knowledge of a secret without disclosing the secret itself. Zero-knowledge proofs have applications in privacy, security, and authentication.
Machine Learning
Machine learning is a field of artificial intelligence where algorithms are used to enable computer systems to learn and make predictions or decisions based on data. It has various applications, including image recognition, natural language processing, and recommendation systems.
Also Read: What is Layer 2 in Blockchain?
ZKML Concept
Zero-knowledge machine learning (ZKML) combines these two domains by leveraging zero-knowledge proofs to enhance the privacy and security of machine learning models and data. In traditional machine learning, the process often involves sharing sensitive data with a centralized server or a third party, raising concerns about privacy and data security.
Privacy Preservation
Zero-knowledge machine learning (ZKML) addresses these concerns by allowing machine learning models to be trained on encrypted data, and the model can make predictions without decrypting the data. The zero-knowledge proof ensures that the model has learned from the data correctly without exposing the data itself. This way, data owners can maintain control over their sensitive information.
Use Cases
Zero-knowledge machine learning (ZKML) can be particularly useful in scenarios where privacy and data security are paramount, such as in healthcare (protecting patient records), financial services (protecting transaction data), and other industries where sensitive data needs to be used for machine learning applications.
Challenges
Implementing Zero-knowledge machine learning (ZKML) can be computationally intensive and may require advanced cryptographic techniques. It’s essential to strike a balance between data privacy and computational efficiency.
The mechanics behind Zero-knowledge machine learning (ZKML) involve cryptographic techniques, secure multiparty computation, and zero-knowledge proofs to ensure that machine learning can be carried out on sensitive data without exposing that data. This concept is still an active area of research and development, with the aim of making machine learning more privacy-preserving and secure.
Why is ZKML a Game-Changer for Privacy?
Zero-Knowledge Machine Learning (ZKML) is indeed a game-changer for privacy because it addresses one of the most significant challenges in the field of data privacy, which is the need to balance the utility of machine learning models with the protection of sensitive data. Here’s a more detailed context on why Zero-knowledge machine learning (ZKML) is so important for privacy:
Preserving Data Privacy
Zero-knowledge machine learning (ZKML) allows machine learning models to be trained on sensitive data without ever exposing that data directly to the model or to any other party involved. This ensures that the data remains confidential and is not at risk of being exposed or misused.
Secure Machine Learning in Sensitive Domains
In fields where privacy is of utmost importance, such as healthcare, finance, and legal, Zero-knowledge machine learning (ZKML) enables organizations to leverage the power of machine learning while adhering to strict privacy regulations and protecting sensitive information. For example, medical records can be used to train predictive models without revealing the individual patient data.
User Control
Zero-knowledge machine learning (ZKML) gives individuals more control over their data. Users can participate in machine learning processes without sharing their data directly. This empowers individuals to engage in services that require machine learning without compromising their privacy.
Compliance with Data Protection Regulations
Zero-knowledge machine learning (ZKML) can help organizations comply with data protection regulations like the General Data Protection Regulation (GDPR) in the European Union. By not exposing raw data, organizations can avoid hefty fines for data breaches and privacy violations.
Business Collaboration
In many cases, organizations can collaborate on machine learning projects without revealing their proprietary data to each other. This is especially beneficial in industries where competitors are looking to jointly develop solutions while safeguarding their intellectual property.
Ethical Considerations
Zero-knowledge machine learning (ZKML) aligns with ethical considerations around data privacy and consent. It reduces the risk of unethical uses of data, and individuals or organizations can give their consent to participate in machine learning projects with a higher level of trust.
Reduced Security Risks
Traditional machine learning models are susceptible to various attacks, such as adversarial attacks and model inversion attacks. Zero-knowledge machine learning (ZKML) reduces these risks, as attackers cannot gain insights into sensitive data, making it more difficult to compromise the model.
Data Monetization
Zero-knowledge machine learning (ZKML) enables data owners to monetize their data without selling or sharing the data itself. This opens up new business models and revenue streams for organizations without compromising data privacy.
Long-Term Privacy Protection
With Zero-knowledge machine learning (ZKML), the privacy of data can be protected not just during training but throughout the model’s lifecycle. This ensures that data remains private even when the model is deployed for predictions and updates.
Public Acceptance
As privacy concerns grow, the use of Zero-knowledge machine learning (ZKML) can enhance public trust in machine learning technologies, fostering greater adoption and acceptance of these technologies.
Zero-Knowledge Machine Learning (ZKML) is a game-changer for privacy as it offers a way to leverage the power of machine learning while ensuring that sensitive data remains private and secure. It has the potential to revolutionize how organizations handle data, promote ethical data practices, and empower individuals to have greater control over their personal information in the digital age.
Real-World Applications of ZKML
Zero-Knowledge Machine Learning (ZKML) is a cutting-edge technology that combines concepts from zero-knowledge proofs and machine learning. In ZKML, a model is trained on sensitive data without the need to expose that data directly. This technology has the potential to revolutionize how we handle sensitive information in various real-world applications. Here are some of the potential real-world applications of Zero-Knowledge Machine Learning:
Medical Research and Diagnostics
Zero-knowledge machine learning (ZKML) can be used to develop predictive models and diagnostic tools without revealing the private health data of individuals. Hospitals and research institutions can collaborate on building robust models while ensuring patient privacy.
Financial Services
Banks and financial institutions can use Zero-knowledge machine learning (ZKML) to build models for fraud detection, risk assessment, and credit scoring without sharing customer-specific financial data. This helps in maintaining the privacy and security of sensitive financial information.
Law Enforcement and Security
Zero-knowledge machine learning (ZKML) can aid in threat detection and security applications without revealing sensitive surveillance data. This is particularly useful for organizations like airports, border security, and public safety agencies.
Smart Cities and Urban Planning
Urban planners can use Zero-knowledge machine learning (ZKML) to create models for traffic management, energy consumption, and other city services without compromising the privacy of residents.
Supply Chain and Inventory Management
Companies can optimize their supply chains and inventory management systems by sharing aggregated data without disclosing individual suppliers’ or customers’ confidential information.
Education
Zero-knowledge machine learning (ZKML) can be used to improve personalized learning systems without exposing student performance data. It enables the development of adaptive learning platforms while maintaining privacy.
Environmental Monitoring
Zero-knowledge machine learning (ZKML) can be applied to analyze and predict climate patterns and environmental changes without revealing sensitive data sources or compromising security.
Market Research and Consumer Insights
Companies can gain valuable market insights without exposing customer profiles or purchasing history. This can lead to more targeted marketing campaigns and product development.
Legal and Compliance
Organizations can use Zero-knowledge machine learning (ZKML) to ensure compliance with privacy regulations, such as GDPR, by minimizing data exposure while still benefiting from data analytics.
Collaborative AI Research
Zero-knowledge machine learning (ZKML) can foster collaboration among organizations and researchers, allowing them to collectively train machine learning models without sharing proprietary datasets, which can be beneficial in scientific research and innovation.
IoT and Smart Devices
In the context of the Internet of Things (IoT), Zero-knowledge machine learning (ZKML) can help protect users’ privacy while still enabling devices to collect and share data for various applications, such as smart homes and health monitoring.
Personalized Healthcare
Zero-knowledge machine learning (ZKML) can be used for creating personalized healthcare recommendations and treatment plans while preserving the privacy of patients’ medical records.
Legal and E-discovery
Zero-knowledge machine learning (ZKML) can facilitate e-discovery processes in legal cases, allowing the extraction of relevant information from documents without revealing the content of sensitive or privileged documents.
Human Resources
Companies can use Zero-knowledge machine learning (ZKML) to enhance HR processes, such as talent recruitment and employee evaluation, while keeping employee data confidential.
Public Health
Zero-knowledge machine learning (ZKML) can be applied to analyze the spread of diseases and epidemiological trends without disclosing individual health information, as seen during the COVID-19 pandemic.
It’s important to note that while Zero-knowledge machine learning (ZKML) offers many promising applications for preserving data privacy, it is a relatively new and evolving field. As it matures, more practical use cases and real-world applications are likely to emerge, potentially transforming various industries by addressing the tension between data utility and privacy concerns.
The Future of ZKML
The field of Zero-Knowledge Machine Learning (ZKML) is in its nascent stages, but it holds tremendous promise for the future. As we look ahead, several key developments and trends can be expected:
Integration with Quantum Computing
One of the most exciting prospects for Zero-knowledge machine learning (ZKML) is its integration with quantum computing. Quantum computers, with their potential for exponential computational power, have the capacity to dramatically accelerate the processing of encrypted data.
This synergy between Zero-knowledge machine learning (ZKML) and quantum computing could unlock entirely new realms of secure, privacy-preserving machine learning applications. For instance, it could enable rapid training of machine learning models on encrypted data, providing solutions to complex problems while preserving user privacy.
Mainstream Adoption
Zero-knowledge machine learning (ZKML) is likely to see a significant surge in adoption as awareness and understanding of its potential benefits spread. Industries across the board, from healthcare to finance, and even governmental agencies, are recognizing the value of preserving data privacy and security in a world awash with sensitive information.
As the technology matures and trust in its capabilities grows, we can anticipate widespread integration of Zero-knowledge machine learning (ZKML) into data processing frameworks. This adoption could lead to new and innovative use cases, such as privacy-preserving AI in healthcare, finance, and more.
Evolution of Standards
The continued development of Zero-knowledge machine learning (ZKML) will be strongly influenced by the establishment of open standards and collaborative research efforts. These standards will be critical in ensuring the security and reliability of Zero-knowledge machine learning (ZKML) solutions in the face of emerging threats and vulnerabilities. The ongoing collaboration among researchers, institutions, and industries will drive the evolution of Zero-knowledge machine learning (ZKML), keeping it adaptable to the changing landscape of cybersecurity and privacy challenges.
Also Read: The Essence of Peer-to-Peer Payments
This collective effort is essential for not only pushing the boundaries of Zero-knowledge machine learning (ZKML) but also for maintaining its resilience and relevance in a dynamic technological environment.
The future of Zero-Knowledge Machine Learning holds immense potential for transforming how we handle sensitive data, ensuring privacy and security in an increasingly data-driven world. With the integration of quantum computing, mainstream adoption, and the evolution of robust standards, Zero-knowledge machine learning (ZKML) is poised to become a cornerstone of secure machine learning, enabling a wide range of applications that protect user privacy while delivering powerful insights and solutions.
Conclusion
Zero-Knowledge Machine Learning (ZKML) is truly a game-changer when it comes to safeguarding our data in today’s data-driven world. In an age where data influences nearly every aspect of our lives, Zero-knowledge machine learning (ZKML) offers a beacon of hope for our privacy concerns.
This innovative approach not only allows us to harness the power of data but also ensures its confidentiality. By embracing Zero-knowledge machine learning (ZKML), we’re not just setting the stage for technological progress, but also for a future where data and privacy can peacefully coexist.
Imagine a world where you can share your data for important tasks, like healthcare or finance, without worrying about it falling into the wrong hands. Zero-Knowledge Machine Learning (ZKML) enables exactly that. As we approach a new era where data-driven decisions shape our world, ZKML’s importance becomes even more clear. It’s not just about technology; it’s about creating a future where data and privacy can work hand in hand.
In this age of constant data-driven decision-making, Zero-Knowledge Machine Learning (ZKML) emerges as a pivotal innovation. It promises to unlock the potential of data while keeping it safe and secure. This means that we can move forward into a future where data and privacy are not at odds, but instead, they can coexist harmoniously. Embracing Zero-knowledge machine learning (ZKML) is not just about technology; it’s about reshaping the way we approach data in our lives.
Disclaimer: The information provided by HeLa Labs in this article is intended for general informational purposes and does not reflect the company’s opinion. It is not intended as investment advice or recommendations. Readers are strongly advised to conduct their own thorough research and consult with a qualified financial advisor before making any financial decisions.
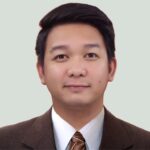
Joshua Soriano
I am Joshua Soriano, a passionate writer and devoted layer 1 and crypto enthusiast. Armed with a profound grasp of cryptocurrencies, blockchain technology, and layer 1 solutions, I've carved a niche for myself in the crypto community.
- Joshua Soriano#molongui-disabled-link
- Joshua Soriano#molongui-disabled-link
- Joshua Soriano#molongui-disabled-link
- Joshua Soriano#molongui-disabled-link